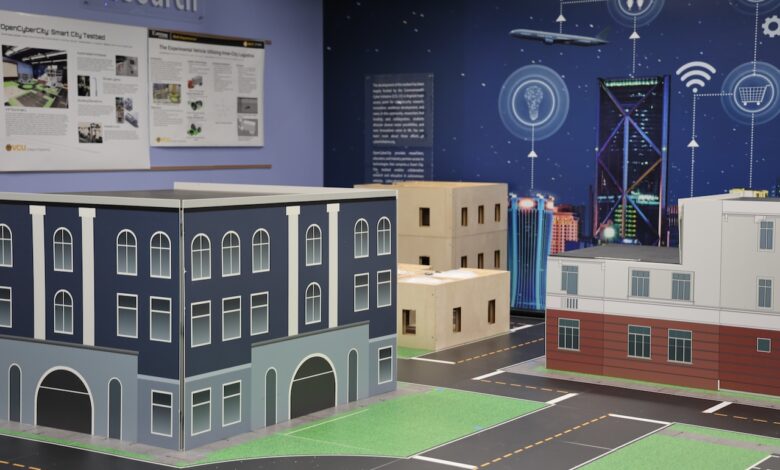
Low Resource Edge Hardware Autoencoders for Anomaly Detection in Smart Cities Networks (LEADS)
PI Jayasimha Atulasimha, Ph.D.
This research aims to develop AI tools for efficient real-time anomaly detection in smart city networks with limited hardware resources.
The researchers address the cybersecurity threats posed by the extensive use of IoT devices in smart cities, emphasizing the need for real-time anomaly detection. To address limited hardware resources at the network’s edge, the research proposes the use of AI tools, specifically autoencoders with simplified synaptic weights, to achieve highly accurate anomaly detection with minimal computational demands.