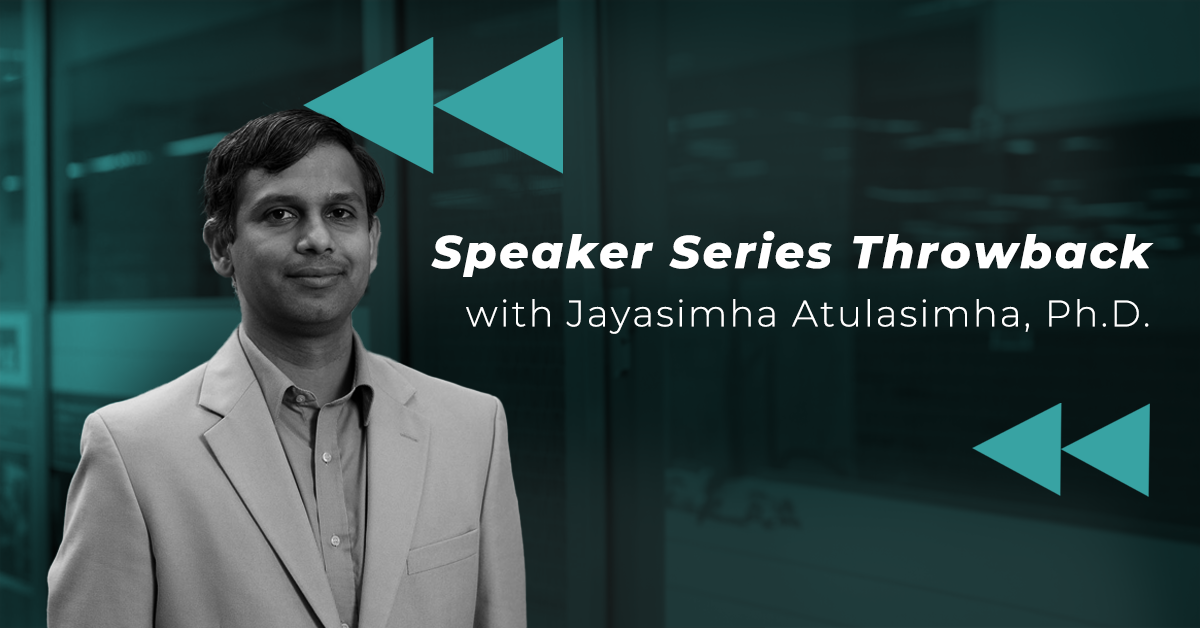
Important research being done by Jayasimha Atulasimha, Ph.D. at Virginia Commonwealth University offers a glimpse into a future where AI hardware takes a proactive stance against cyberattacks, while also addressing the ever-growing concern of energy consumption in AI applications.
The Drive for Efficiency
The motivation behind the research stems from the vast amount of energy required for traditional AI models. Dr. Atulasimha highlights this concern by mentioning that the annual energy consumption for Google LLC alone in 2019 was comparable to a small country’s energy needs. With AI becoming increasingly widespread, this energy requirement for computing will increase exponentially, posing a significant challenge in meeting our world’s computational needs in a sustainable way.
This is also very crucial for edge AI applications, where devices have limited power and rely on batteries. Edge devices collect data from sensors and process it locally, offering faster response times, lower latency, and improved privacy. However, their small size and reliance on batteries necessitates energy-efficient solutions.
Addressing the Challenge: Three Promising Projects
Dr. Atulasimha’s group tackles this challenge through four projects aimed at developing energy-efficient AI hardware for edge devices.
- Quantized Synaptic Weights: This project focuses on reducing the amount of memory needed to store weights by using coarse (less accurate) weights that need to be trained in various neural networks. By quantizing the weights, the researchers can significantly reduce the hardware resources and energy needed to operate such neural networks.
- Reservoir Computing This project explores using reservoir computing, a technique adept at training complex models with limited resources, for anomaly detection at the edge, especially for time series data.
- Quantized Transformers for Building Energy Forecasting: Smart cities rely on building energy forecasting to optimize energy usage. This project investigates the use of quantized transformers, a type of neural network well-suited for processing time series data from building sensors. By quantizing the transformers, Dr. Atulasimha’s group aims to create an energy-efficient solution for smart city applications.
The Road Ahead
Dr. Atulasimha’s research paves the way for a future where AI hardware is not only powerful but also energy-efficient, particularly for edge devices. His exploration of techniques like quantized synaptic weights, reservoir computing, and quantized transformers holds immense potential for making AI more sustainable and enabling novel applications at the edge. This will not only revolutionize cybersecurity but also empower various sectors that rely on edge AI for efficient operations.
Watch Jayasimha Atulasimha Ph. D.’s talk on quantized transformers here.